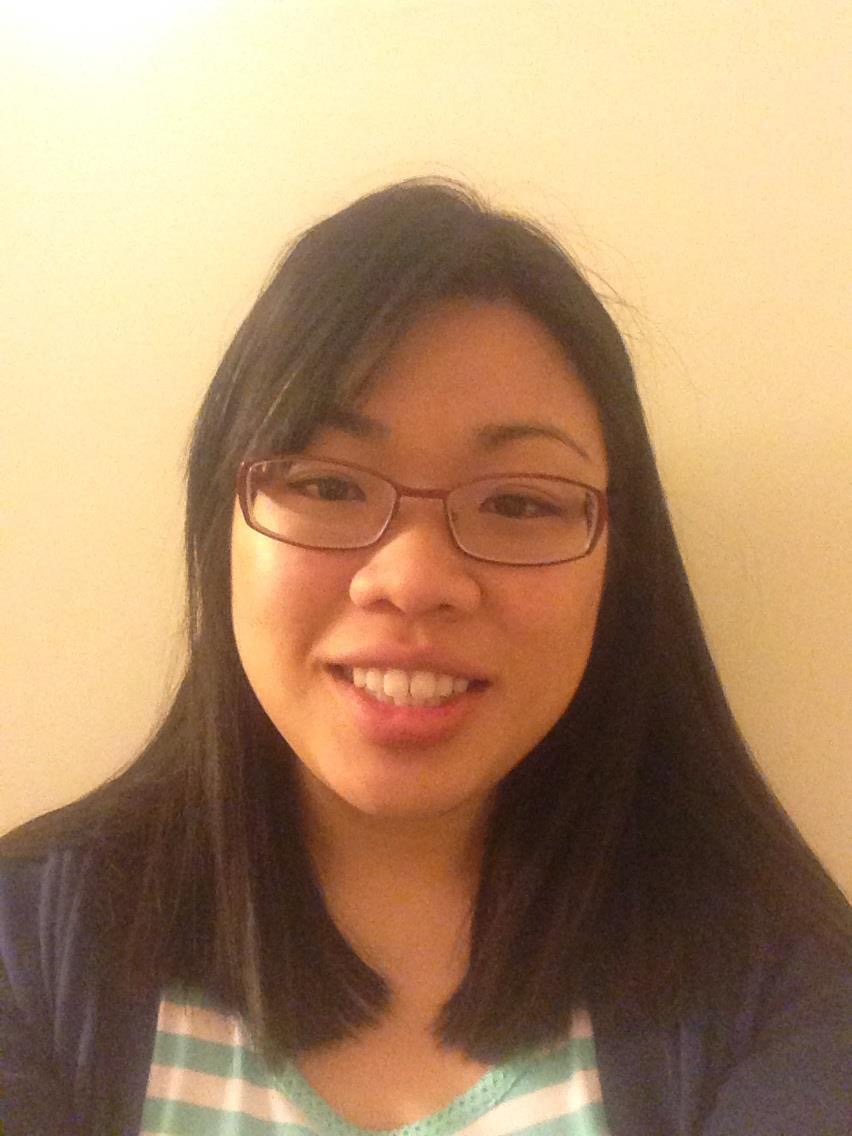
Vivian Duong
December 30, 2018
Predicting Housing Prices 011 Data Prep Outlier
Hunting for Outliers
import numpy as np
import pandas as pd
import warnings; warnings.filterwarnings('ignore')
import matplotlib.pyplot as plt
import seaborn as sns
%matplotlib inline
import pickle
import lib.clean_helper as cl
import lib.viz_helper as vz
train = pd.read_pickle('../data/to_clean_1_train.p')
Tukey Outliers
Using Tukey’s method is not very helpful. It is too sensitive
is_dtype_num = train.dtypes.isin([np.dtype('float64'), np.dtype('int64')])
num_feats = train.dtypes.index[is_dtype_num]
num_df = train[num_feats].copy()
def is_tukey_outlier(column):
q1 = column.quantile(0.25); q3 = column.quantile(0.75)
iqr = q3 - q1
is_outside_window = column.apply(lambda x: x < q1 - 1.5*iqr or x > q3 + 1.5*iqr )
return is_outside_window
num_df['is_t_outlier'] = num_df.apply(is_tukey_outlier).any(axis = 'columns')
for i, col in enumerate(num_df):
sns.lmplot(col,
'SalePrice',
data=num_df,
hue = 'is_t_outlier',
scatter_kws={'alpha': 0.2},
fit_reg = False
)
Eyeballing Works
Sometimes simple “eyeballing” works best. There are outliers where the square footage is high but the prices are low. They’re really clear in GrLivArea
. Perhaps those are the same observations where the features describe square footage are low.
sns.regplot('GrLivArea',
num_df['SalePrice'],
data=num_df,
scatter_kws={'alpha': 0.2}
)
<matplotlib.axes._subplots.AxesSubplot at 0x1e3ca204828>
num_df[num_df.GrLivArea > 4600]
num_df['is_outlier'] = False
num_df.is_outlier[num_df.GrLivArea > 4600] = True
for i, col in enumerate(num_df):
sns.lmplot(col,
'SalePrice',
data=num_df,
hue = 'is_outlier',
scatter_kws={'alpha': 0.2},
fit_reg = False
)
train = train[train.GrLivArea < 4600]
train.to_pickle('../data/to_clean_2_train.p')